Abstract
With the help of traffic information of the connected environment, an energy management strategy (EMS) is proposed based on preceding vehicle speed prediction, host vehicle speed planning, and dynamic programming (DP) with PI correction to improve the fuel economy of connected hybrid electric vehicles (HEVs). A conditional linear Gaussian (CLG) model for estimating the future speed of the preceding vehicle is established and trained by utilizing historical data. Based on the predicted information of the preceding vehicle and traffic light status, the speed curve of the host vehicle can ensure that the vehicle follows safety and complies with traffic rules simultaneously as planned. The real-time power allocation is composed of offline optimization results of DP and the real-time PI correction items according to the actual operation of the engine. The effectiveness of the control strategy is verified by the simulation system of HEVs in the interconnected environment established by E-COSM 2021 on the MATLAB/Simulink and CarMaker platforms.
























Similar content being viewed by others
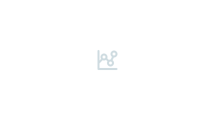
References
Zhang, J., Shen, T., & Kako, J. (2020). Short-term optimal energy management of power-split hybrid electric vehicles under velocity tracking control. IEEE Transactions on Vehicular Technology, 69(1), 182–193. https://doi.org/10.1109/TVT.2019.2950042
Lin, C., Peng, H., & Grizzle, J. (2003). Power management strategy for a parallel hybrid electric truck. IEEE Transactions on Control Systems Technology, 11(6), 839–849. https://doi.org/10.1109/TCST.2003.815606
Sciarretta, A., Serrao, L., & Dewangan, P. (2014). A control benchmark on the energy management of a plug-in hybrid electric vehicle. Control Engineering Practice, 29, 287–298.
Guanetti, J., Kim, Y., & Borrelli, F. (2018). Control of connected and automated vehicles: State of the art and future challenges. Annual Reviews in Control, 45, 18–40. https://doi.org/10.1016/j.arcontrol.2018.04.011
Zhang, J., & Xu, F. (2020). Real-time optimization of energy consumption under adaptive cruise control for connected HEVs. Control Theory and Technology, 18(2), 182–192. https://doi.org/10.1007/s11768-020-0020-7
Moser, D., Schmied, R., & Waschl, H. (2018). Flexible spacing adaptive cruise control using stochastic model predictive control. IEEE Transactions on Control Systems Technology, 26(1), 114–127. https://doi.org/10.1109/TCST.2017.2658193
Pu, Z., Jiao, X., & Yang, C. (2020). An adaptive stochastic model predictive control strategy for plug-in hybrid electric bus during vehicle-following scenario. IEEE Access, 8, 13887–13897. https://doi.org/10.1109/ACCESS.2020.2966531
Nishio, Y., & Shen, T. (2020). A two-stage optimization scheme of fuel consumption and drivability for plug-in HEVs. SICE Journal of Control Measurement and System Integration, 13, 173–182. https://doi.org/10.9746/jcmsi.13.173
Lin, X., Zhang, G., & Wei, S. (2021). Velocity prediction using markov chain combined with driving pattern recognition and applied to dual-motor electric vehicle energy consumption Evaluation. IEEE Applied Soft Computing Journal, 101, 1–14. https://doi.org/10.1016/j.asoc.2020.106998
Zhang, Z., He, H., & Guo, J. (2020). Velocity prediction and profile optimization based real-time energy management strategy for Plug-in hybrid electric buses. Applied Energy, 280, 1–14. https://doi.org/10.1016/j.apenergy.2020.116001
Fu, Q., Xu, F., & Shen, T. (2020). Distributed optimal energy consumption control of HEVs under MFG-based speed consensus. Control Theory and Technology, 18(2), 193–203. https://doi.org/10.1007/s11768-020-0021-6
Singh, K., Bansal, H., & Singh, D. (2021). Fuzzy logic and elman neural network tuned energy management strategies for a power-split HEVs. Energy, 225, 120152. https://doi.org/10.1016/j.energy.2021.120152
Nishio, Y., & Shen, T. (2021). Hybrid deep reinforcement learning based eco-driving for low-level connected and automated vehicles along signalized corridors. Transportation Research Part C: Emerging Technologies, 124, 102980. https://doi.org/10.1016/j.trc.2021.102980
Hu, X., Zhang, X., & Tang, X. (2020). Model predictive control of hybrid electric vehicles for fuel economy, emission reductions, and inter-vehicle safety in car-following scenarios. Energy, 196, 117101. https://doi.org/10.1016/j.energy.2020.117101
Zhang, B., Cao, W., & Shen, T. (2019). Two-stage on-board optimization of merging velocity planning with energy management for HEVs. Control Theory and Technology, 17(4), 335–345. https://doi.org/10.1007/s11768-019-9129-y
Shi, D., Liu, S., & Cai, Y. (2021). Pontryagin’s minimum principle based fuzzy adaptive energy management for hybrid electric vehicle using real-time traffic information. Applied Energy, 286, 116467. https://doi.org/10.1016/j.apenergy.2021.116467
Li, X., Evangelou, S., & Lot, R. (2018) Integrated management of powertrain and engine cooling system for parallel hybrid electric vehicles. IEEE Vehicle Power and Propulsion Conference. Chicago, IL, USA. https://doi.org/10.1109/VPPC.2018.8604994.
Li, P., Jiao, X., & Li, Y. (2020). Adaptive real-time energy management control strategy based on fuzzy inference system for plug-in hybrid electric vehicles. Control Engineering Practice, 107, 104703. https://doi.org/10.1016/j.conengprac.2020.104703
Jeoung, H., Lee, K., & Kim, N. (2019). Methodology for finding maximum performance and improvement possibility of rule-based control for parallel type-2 hybrid electric vehicles. Energies, 12, 1924. https://doi.org/10.3390/en12101924
Biswas, A., & Emadi, A. (2019). Energy management systems for electrified powertrains: State-of-the-art review and future trends. IEEE Transactions on Vehicular Technology, 68, 6453–6467. https://doi.org/10.1109/TVT.2019.2914457
Martnez, C., Hu, X., & Cao, D. (2017). Energy management in plug-in hybrid electric vehicles: Recent progress and a connected vehicles perspective. IEEE Transactions on Vehicular Technology, 66, 4534–4549. https://doi.org/10.1109/TVT.2016.2582721
Ma, F., Yang, Y., & Wang, J. (2019). Predictive energy-saving optimization based on nonlinear model predictive control for cooperative connected vehicles platoon with V2V communication. Energy, 189, 116120. https://doi.org/10.1016/j.energy.2019.116120
Ding, J., Li, L., & Peng, H. (2020). A rule-based cooperative merging strategy for connected and automated vehicles. IEEE Transactions on Intelligent Transportation System, 21, 3436–3446. https://doi.org/10.1109/TITS.2019.2928969
Asadi, B., & Vahidi, A. (2011). Predictive cruise control: utilizing upcoming traffic signal information for improving fuel economy and reducing trip time. IEEE Transactions on Control Systems Technology, 19, 707–714. https://doi.org/10.1109/TCST.2010.2047860
Xu, F., Tsunogawa, H., & Kako, J. (2020). Real-time energy optimization of HEVs under connected environment: ECOSM 2021 benchmark problem and a aase study. EnerarXiv-preprint. https://www.enerarxiv.org/page/thesis.html?id=2006.
Author information
Authors and Affiliations
Corresponding author
Additional information
This work was supported by the National Natural Science Foundation of China (No. 61973265), the Natural Science Foundation of Hebei Province (No. E2021203079) and the Scientific Research Foundation for the Returned Overseas Chinese Scholars, Hebei Province (No. C20210323)
Rights and permissions
About this article
Cite this article
You, X., Jiao, X., Wei, Z. et al. Real-time energy management strategy based on predictive cruise control for hybrid electric vehicles. Control Theory Technol. 20, 161–172 (2022). https://doi.org/10.1007/s11768-022-00096-w
Received:
Revised:
Accepted:
Published:
Issue Date:
DOI: https://doi.org/10.1007/s11768-022-00096-w