This Paper:Browse 873 Download 103 |
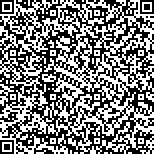 码上扫一扫! |
Class conditional distribution alignment for domain adaptation |
KaiCao,ZhipengTu,YangMing |
|
(Key Lab of Systems and Control, Academy of Mathematics and Systems Science, Chinese Academy of Sciences, Beijing 100190, China; University of Chinese Academy of Sciences, Beijing 100190, China) |
|
摘要: |
In this paper, we study the problem of domain adaptation, which is a crucial ingredient in transfer learning with two domains, that is, the source domain with labeled data and the target domain with none or few labels. Domain adaptation aims to extract knowledge from the source domain to improve the performance of the learning task in the target domain. A popular approach to handle this problem is via adversarial training, which is explained by the $\mathcal H \Delta \mathcal H$-distance theory. However, traditional adversarial network architectures just align the marginal feature distribution in the feature space. The alignment of class condition distribution is not guaranteed. Therefore, we proposed a novel method based on pseudo labels and the cluster assumption to avoid the incorrect class alignment in the feature space. The experiments demonstrate that our framework improves the accuracy on typical transfer learning tasks. |
关键词: Domain adaptation, distribution alignment, feature cluster |
DOI:https://doi.org/10.1007/s11768-020-9126-1 |
|
基金项目:This work was supported by the National Key Research and Development Program of China (No. 2016YFB0901902) and the National Natural Science Foundation of China (No. 61733018). |
|
Class conditional distribution alignment for domain adaptation |
Kai Cao,Zhipeng Tu,Yang Ming |
(Key Lab of Systems and Control, Academy of Mathematics and Systems Science, Chinese Academy of Sciences, Beijing 100190, China; University of Chinese Academy of Sciences, Beijing 100190, China) |
Abstract: |
In this paper, we study the problem of domain adaptation, which is a crucial ingredient in transfer learning with two domains, that is, the source domain with labeled data and the target domain with none or few labels. Domain adaptation aims to extract knowledge from the source domain to improve the performance of the learning task in the target domain. A popular approach to handle this problem is via adversarial training, which is explained by the $\mathcal H \Delta \mathcal H$-distance theory. However, traditional adversarial network architectures just align the marginal feature distribution in the feature space. The alignment of class condition distribution is not guaranteed. Therefore, we proposed a novel method based on pseudo labels and the cluster assumption to avoid the incorrect class alignment in the feature space. The experiments demonstrate that our framework improves the accuracy on typical transfer learning tasks. |
Key words: Domain adaptation, distribution alignment, feature cluster |