This Paper:Browse 566 Download 0 |
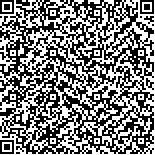 码上扫一扫! |
Improving the classification accuracy using biomarkers selected from machine learning methods |
LinduniM.Rodrigo,AshokaD.Polpitiya |
|
(1 Department of Mathematics and Statistics, University of Sydney, Sydney, NSW 2006, Australia;2 Colombo School of Business and Management, Colombo, Sri Lanka) |
|
摘要: |
High-dimensional data encountered in genomic and proteomic studies are often limited by the sample size but has a higher
number of predictor variables. Therefore selecting the most relevant variables that are correlated with the outcome variable
is a crucial step. This paper describes an approach for selecting a set of optimal variables to achieve a classification model
with high predictive accuracy. The work described using a biological classifier published elsewhere but it can be generalized
for any application. |
关键词: Classification · Variable selection · Reversal · Regression |
DOI:https://doi.org/10.1007/s11768-021-00071-x |
|
基金项目: |
|
Improving the classification accuracy using biomarkers selected from machine learning methods |
Linduni M. Rodrigo,Ashoka D. Polpitiya |
(1 Department of Mathematics and Statistics, University of Sydney, Sydney, NSW 2006, Australia;2 Colombo School of Business and Management, Colombo, Sri Lanka) |
Abstract: |
High-dimensional data encountered in genomic and proteomic studies are often limited by the sample size but has a higher
number of predictor variables. Therefore selecting the most relevant variables that are correlated with the outcome variable
is a crucial step. This paper describes an approach for selecting a set of optimal variables to achieve a classification model
with high predictive accuracy. The work described using a biological classifier published elsewhere but it can be generalized
for any application. |
Key words: Classification · Variable selection · Reversal · Regression |