This Paper:Browse 378 Download 0 |
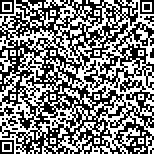 码上扫一扫! |
Characterizing human driver characteristics using an artificial neural network and a theoretical model |
SangmyoengKim1,TakeshiMiyamoto1,TatsuyaKuboyama1,YasuoMoriyoshi1 |
|
(1 Graduate School of Engineering, Chiba University, 1-33 Yayoicho Inageku, Chiba 2638522, Japan) |
|
摘要: |
Human drivers seem to have different characteristics, so different drivers often yield different results from the same driving
mode tests with identical vehicles and same chassis dynamometer. However, drivers with different experiences often yield
similar results under the same driving conditions. If the features of human drivers are known, the control inputs to each driver,
including warnings, will be customized to optimize each man–machine vehicle system. Therefore, it is crucial to determine
how to characterize human drivers quantitatively. This study proposes a method to estimate the parameters of a theoretical
model of human drivers. Themethod uses an artificial neural network (ANN) model and a numerical procedure to interpret the
identified ANN models theoretically. Our approach involves the following process. First, we specify each ANN driver model
through chassis dynamometer tests performed by each human driver and vehicle. Subsequently, we obtain the parameters of
a theoretical driver model using the ANN model for the corresponding driver. Specifically, we simulate the driver’s behaviors
using the identified ANN models with controlled inputs. Finally, we estimate the theoretical driver model parameters using
the numerical simulation results. A proportional-integral-differential (PID) control model is used as the theoretical model.
The results of the parameter estimation indicate that the PID driver model parameter combination can characterize human
drivers. Moreover, the results suggest that vehicular factors influence the parameter combinations of human drivers. |
关键词: Neural network · Identification · Driver model · Chassis dynamometer · Driver characteristics |
DOI:https://doi.org/10.1007/s11768-022-00099-7 |
|
基金项目: |
|
Characterizing human driver characteristics using an artificial neural network and a theoretical model |
Sangmyoeng Kim1,Takeshi Miyamoto1,Tatsuya Kuboyama1,Yasuo Moriyoshi1 |
(1 Graduate School of Engineering, Chiba University, 1-33 Yayoicho Inageku, Chiba 2638522, Japan) |
Abstract: |
Human drivers seem to have different characteristics, so different drivers often yield different results from the same driving
mode tests with identical vehicles and same chassis dynamometer. However, drivers with different experiences often yield
similar results under the same driving conditions. If the features of human drivers are known, the control inputs to each driver,
including warnings, will be customized to optimize each man–machine vehicle system. Therefore, it is crucial to determine
how to characterize human drivers quantitatively. This study proposes a method to estimate the parameters of a theoretical
model of human drivers. Themethod uses an artificial neural network (ANN) model and a numerical procedure to interpret the
identified ANN models theoretically. Our approach involves the following process. First, we specify each ANN driver model
through chassis dynamometer tests performed by each human driver and vehicle. Subsequently, we obtain the parameters of
a theoretical driver model using the ANN model for the corresponding driver. Specifically, we simulate the driver’s behaviors
using the identified ANN models with controlled inputs. Finally, we estimate the theoretical driver model parameters using
the numerical simulation results. A proportional-integral-differential (PID) control model is used as the theoretical model.
The results of the parameter estimation indicate that the PID driver model parameter combination can characterize human
drivers. Moreover, the results suggest that vehicular factors influence the parameter combinations of human drivers. |
Key words: Neural network · Identification · Driver model · Chassis dynamometer · Driver characteristics |