This Paper:Browse 294 Download 0 |
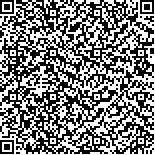 码上扫一扫! |
Random gradient-free method for online distributed optimization with strongly pseudoconvex cost functions |
XiaoxiYan1,ChengLi1,KaihongLu2,HangXu2 |
|
(1 School of Electrical and Information Engineering, Jiangsu University, Zhenjiang 212013, Jiangsu, China;;2 College of Electrical Engineering and Automation, Shandong University of Science and Technology, Qingdao 266590, Shandong, China.) |
|
摘要: |
This paper focuses on the online distributed optimization problem based on multi-agent systems. In this problem, each agent
can only access its own cost function and a convex set, and can only exchange local state information with its current neighbors
through a time-varying digraph. In addition, the agents do not have access to the information about the current cost functions
until decisions are made. Different from most existing works on online distributed optimization, here we consider the case
where the cost functions are strongly pseudoconvex and real gradients of the cost functions are not available. To handle this
problem, a random gradient-free online distributed algorithm involving the multi-point gradient estimator is proposed. Of
particular interest is that under the proposed algorithm, each agent only uses the estimation information of gradients instead
of the real gradient information to make decisions. The dynamic regret is employed to measure the proposed algorithm. We
prove that if the cumulative deviation of the minimizer sequence grows within a certain rate, then the expectation of dynamic
regret increases sublinearly. Finally, a simulation example is given to corroborate the validity of our results. |
关键词: Multi-agent system · Online distributed optimization · Pseudoconvex optimization · Random gradient-free method |
DOI:https://doi.org/10.1007/s11768-023-00181-8 |
|
基金项目:This work was supported by the National Natural Science Foundation of China (Nos. 62103169, 51875380) and the China Postdoctoral Science Foundation (No. 2021M691313). |
|
Random gradient-free method for online distributed optimization with strongly pseudoconvex cost functions |
Xiaoxi Yan1,Cheng Li1,Kaihong Lu2,Hang Xu2 |
(1 School of Electrical and Information Engineering, Jiangsu University, Zhenjiang 212013, Jiangsu, China;;2 College of Electrical Engineering and Automation, Shandong University of Science and Technology, Qingdao 266590, Shandong, China.) |
Abstract: |
This paper focuses on the online distributed optimization problem based on multi-agent systems. In this problem, each agent
can only access its own cost function and a convex set, and can only exchange local state information with its current neighbors
through a time-varying digraph. In addition, the agents do not have access to the information about the current cost functions
until decisions are made. Different from most existing works on online distributed optimization, here we consider the case
where the cost functions are strongly pseudoconvex and real gradients of the cost functions are not available. To handle this
problem, a random gradient-free online distributed algorithm involving the multi-point gradient estimator is proposed. Of
particular interest is that under the proposed algorithm, each agent only uses the estimation information of gradients instead
of the real gradient information to make decisions. The dynamic regret is employed to measure the proposed algorithm. We
prove that if the cumulative deviation of the minimizer sequence grows within a certain rate, then the expectation of dynamic
regret increases sublinearly. Finally, a simulation example is given to corroborate the validity of our results. |
Key words: Multi-agent system · Online distributed optimization · Pseudoconvex optimization · Random gradient-free method |